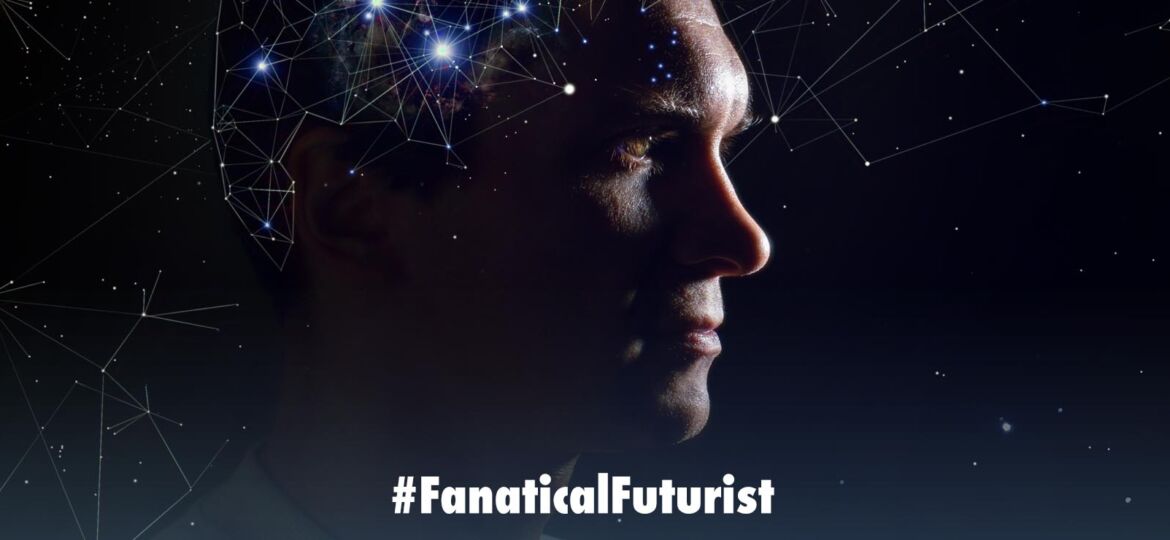
WHY THIS MATTERS IN BRIEF
We’re conditioned to think that computer chips should be small, but a supercomputer made from chips the size of dinner plates is breaking records.
Love the Exponential Future? Join our XPotential Community, future proof yourself with courses from XPotential University, read about exponential tech and trends, connect, watch a keynote, or browse my blog.
Artificial Intelligence (AI) is on a tear. Machines can speak, write, play games, and generate original images, video, and music. But as AI’s capabilities have grown, so too have the size of its algorithms. A decade ago, machine learning algorithms relied on tens of millions of internal connections, or parameters. Today’s algorithms regularly reach into the hundreds of billions and even trillions of parameters. Researchers say scaling up still yields performance gains, and models with tens of trillions of parameters may arrive in short order.
To train models that big, you need powerful computers. Whereas AI in the early 2010s ran on a handful of Graphics Processing Units (GPU) – computer chips that excel at the parallel processing crucial to AI – computing needs have grown exponentially, and top models now require hundreds or thousands of GPUs. As a result companies such as OpenAI, Microsoft, Meta, and others are building dedicated supercomputers, or in Microsoft’s case turning their Azure cloud infrastructure into the world’s largest distributed supercomputer, to handle the task, and they say these AI machines rank among the fastest on the planet.
The Future of Artificial Intelligence, by keynote speaker Matthew Griffin
But even as GPUs have been crucial to AI scaling – Nvidia’s A100, for example, is still one of the fastest, most commonly used chips in AI clusters – weirder alternatives designed specifically for AI have popped up in recent years. Enter Cerebras.
The size of a dinner plate – about 8.5 inches to a side with over 2.6 Trillion transistors each – the company’s Wafer Scale Engine is the biggest silicon chip in the world, boasting 2.6 trillion transistors and 850,000 cores etched onto a single silicon wafer. Each Wafer Scale Engine serves as the heart of the company’s CS-2 computer.
Alone, the CS-2 is a beast, but last year Cerebras unveiled a plan to link CS-2s together with an external memory system called MemoryX and a system to connect CS-2s called SwarmX. The company said the new tech could link up to 192 chips and train models two orders of magnitude larger than today’s biggest, most advanced AIs.
“The industry is moving past 1-trillion-parameter models, and we are extending that boundary by two orders of magnitude, enabling brain-scale neural networks with 120 trillion parameters,” Cerebras CEO and cofounder Andrew Feldman said.
At the time, all this was theoretical. But last week, the company announced they’d linked 16 CS-2s together into a world-class AI supercomputer.
The new machine, called Andromeda, has 13.5 million cores capable of speeds over an exaflop, or one quintillion operations per second, at 16-bit half precision. Due to the unique chip at its core, Andromeda isn’t easily compared to supercomputers running on more traditional CPUs and GPUs, but Feldman told HPC Wire Andromeda is roughly equivalent to Argonne National Laboratory’s Polaris supercomputer, which ranks 17th fastest in the world, according to the latest Top500 list.
In addition to performance, Andromeda’s speedy build time, cost, and footprint are notable. Argonne began installing Polaris in the summer of 2021, and the supercomputer went live about a year later. It takes up 40 racks, the filing-cabinet-like enclosures housing supercomputer components. By comparison, Andromeda cost $35 million – a modest price for a machine of its power – took just three days to assemble, and uses a mere 16 racks.
Cerebras tested the system by training five versions of OpenAI’s large language model GPT-3 as well as Eleuther AI’s open source GPT-J and GPT-NeoX. And according to Cerebras, perhaps the most important finding is that Andromeda demonstrated what they call “near-perfect linear scaling” of AI workloads for large language models. In short, that means as additional CS-2s are added, training times decrease proportionately.
Typically, the company said, as you add more chips, performance gains diminish. Cerebras’s WSE chip, on the other hand, may prove to scale more efficiently because its 850,000 cores are connected to each other on the same piece of silicon. What’s more, each core has a memory module right next door. Taken together, the chip slashes the amount of time spent shuttling data between cores and memory.
“Linear scaling means when you go from one to two systems, it takes half as long for your work to be completed. That is a very unusual property in computing,” Feldman told HPC Wire. And, he said, it can scale beyond 16 connected systems.
Beyond Cerebras’s own testing, the linear scaling results were also demonstrated during work at Argonne National Laboratory where researchers used Andromeda to train the GPT-3-XL large language algorithm on long sequences of the Covid-19 genome.
Of course, though the system may scale beyond 16 CS-2s, to what degree linear scaling persists remains to be seen. Also, we don’t yet know how Cerebras performs head-to-head against other AI chips. AI chipmakers like Nvidia and Intel have begun participating in regular third-party benchmarking by the likes of MLperf. Cerebras has yet to take part.
Still, the approach does appear to be carving out its own niche in the world of supercomputing, and continued scaling in large language AI is a prime use case. Indeed, Feldman told Wired last year that the company was already talking to engineers at OpenAI, a leader in large language models, and coincidentally OpenAI founder, Sam Altman, is also an investor in Cerebras.
On its release in 2020, OpenAI’s large language model GPT-3, changed the game both in terms of performance and size. Weighing in at 175 billion parameters, it was the biggest AI model at the time and surprised researchers with its abilities. Since then, language models have reached into the trillions of parameters, and larger models may be forthcoming. There are rumors – just that, so far – that OpenAI will release GPT-4 in the not-too-distant future and it will be another leap from GPT-3.
That said, despite their capabilities, large language models are neither perfect nor universally adored. Their flaws include output that can be false, biased, and offensive. Meta’s Galactica, trained on scientific texts, is a recent example. Despite a dataset one might assume is less prone to toxicity than training on the open internet, the model was easily provoked into generating harmful and inaccurate text and pulled down in just three days. Whether researchers can solve language AI’s shortcomings remains uncertain.
But it seems likely that scaling up will continue until diminishing returns kick in. The next leap could be just around the corner, and we may already have the hardware to make it happen.