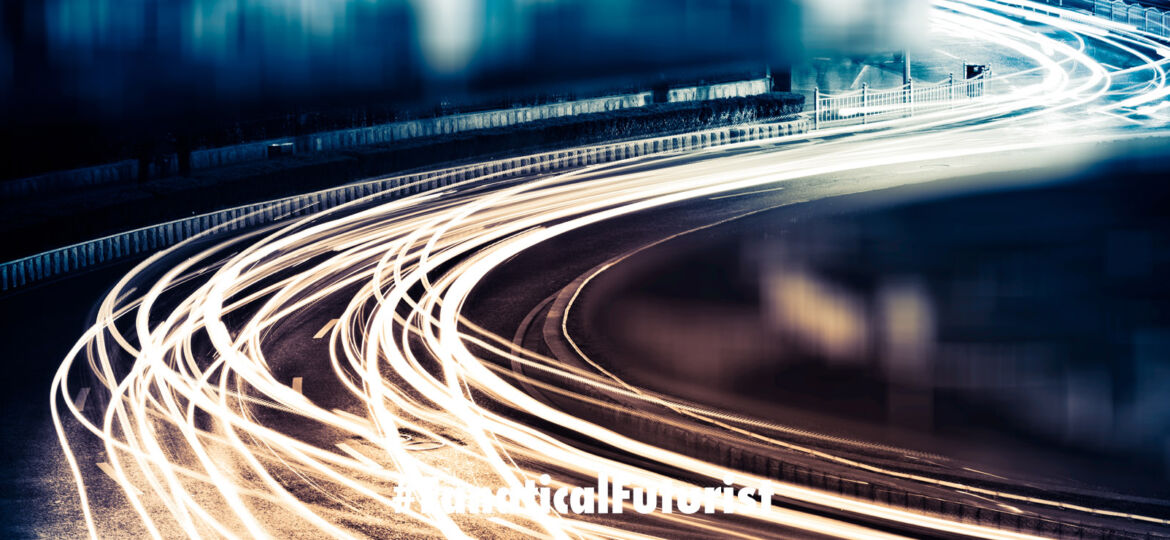
WHY THIS MATTERS IN BRIEF
As AI becomes both the tool and the creator of graphics and content, you can expect to see much more of this in the future.
Interested in the Exponential Future? Connect, download a free E-Book, watch a keynote, or browse my blog.
The recent boom in Artificial Intelligence (AI) has led to the emergence of an entirely new field of research dedicated to using AI’s, or Creative Machines as they’re also known, to create synthetic content – or in laymans terms create “fake” digital content without the involvement of humans that includes everything from audio tracks, and imagery, to videos.
As part of this new trend I recently reported how Promethean AI was using its AI to help people create game environments just by talking and describing what they wanted, and how Nvidia had created an AI that could take in a real video feed, from a city for example, and transform it in real time into digital content that could be used to create game environments as well as VR worlds. And at the time both of these were huge breakthroughs in the field.
Now, in their latest research the same team behind the original Nvidia breakthrough have published research showing how AI generated video and visuals can be combined with a traditional video game engine “to create a hybrid graphics system” that could one day be used in video games, movies, and virtual reality.
“It’s a new way to render [and generate] video content using deep learning,” said Nvidia’s vice president of applied deep learning, Bryan Catanzaro. “Obviously Nvidia cares a lot about generating graphics [and] we’re thinking about how AI is going to revolutionize the field.”
The results of Nvidia’s work aren’t photorealistic though and show the trademark visual smearing found in much AI generated imagery. In a research paper, the company’s engineers explain how they built upon a number of existing methods, including an influential open-source system called pix2pix. Their works uses a type of neural network known as a Generative Adversarial Network, or GAN. These are widely used in AI image generation, including for the creation of an AI portrait that was recently sold by Christie’s for over $400,000, as well as the first Text to Video clips and much more.
The new technique has also helped the team achieve another world first, the company says, by creating the first ever video game demo using only AI generated graphics. The demo’s a simple driving simulator where players navigate a few city blocks of AI generated space, but can’t leave their car or otherwise interact with the world, and the demo is powered using just a single GPU — a notable achievement for such cutting-edge work.
Nvidia’s system generates graphics using just a few steps. First, researchers have to collect training data, which in this case was taken from open-source datasets used for autonomous driving research. This footage is then segmented, meaning each frame is broken into different categories: sky, cars, trees, road, buildings, and so on. A GAN is then trained on this segmented data to generate new digital versions of these objects.
Next, engineers created the basic topology of the virtual environment using a traditional game engine. In this case the system was Unreal Engine 4, a popular engine used for titles such as Fortnite, PUBG, Gears of War 4, and many others. Using this environment as a framework, deep learning algorithms then generated the graphics for each different category of item in real time, pasting them on to the game engine’s models.
“The structure of the world is being created traditionally,” explains Catanzaro, “the only thing the AI generates is the graphics.” He adds that the demo itself is basic, and was put together by a single engineer. “It’s proof-of-concept rather than a game that’s fun to play.”
To create this system Nvidia’s engineers had to work around a number of challenges, the biggest of which was object permanence. The problem is, if the deep learning algorithms are generating the graphics for the world at a rate of 25 frames per second, how do they keep objects looking the same? Catanzaro says this problem meant the initial results of the system were “painful to look at” as colors and textures “changed in every frame.”
The solution was to give the system a short-term memory, a little like the one Google DeepMind created for their own state of the art AI’s, so that it would compare each new frame with what’s gone before. It tries to predict things like motion within these images, and creates new frames that are consistent with what’s on screen. All this computation is expensive though, and so the game only runs at 25 frames per second.
The technology is very much at the early stages, stresses Catanzaro, and it will likely be many years until AIgenerated graphics show up in consumer titles. He compares the situation to the development of ray tracing, the current hot technique in graphics rendering where individual rays of light are generated in real time to create realistic reflections, shadows, and opacity in virtual environments.
“The very first interactive ray tracing demo happened a long, long time ago, but we didn’t get it in games [such as Minecraft] until just a few weeks ago,” he says.
The work does have potential applications in other areas of research, though, including robotics simulators and self-driving cars, where it could be used to generate training environments like the one Amazon used recently to train their Scout drone. And it could show up in consumer products sooner albeit in a more limited capacity.
For example, this technology could be used in a hybrid graphics system, where the majority of a game is rendered using traditional methods, but AI is used to create the likenesses of people or objects. Consumers could capture footage themselves using smartphones, then upload this data to the cloud where algorithms would learn to copy it and insert it into games. It would make it easier to create avatars that look just like players, for example.
This sort of technology raises some obvious questions, though. In recent years experts have become increasingly worried about the use of AI-generated deepfakes for disinformation and propaganda. Researchers have shown it’s easy to generate fake footage of politicians and celebrities saying or doing things that they didn’t, a potent weapon in the wrong hands. By pushing forward the capabilities of this technology and publishing its research, Nvidia is arguably contributing to this potential problem. The company, though, says this is hardly a new issue.
“Can [this technology] be used for creating content that’s misleading? Yes. Any technology for rendering can be used to do that,” says Catanzaro. He says Nvidia is working with partners to research methods for detecting AI fakes, but that ultimately the problem of misinformation is a “trust issue.” And, like many trust issues before it, it will have to be solved with an array of methods, not just technological.
Catanzaro says tech companies like Nvidia can only take so much responsibility.
“Do you hold the power company responsible because they created the electricity that powers the computer that makes the fake video?” he asks.
And ultimately, for Nvidia, pushing forward with AI-generated graphics has an obvious benefit – it’ll help sell more of the company’s hardware. Since the deep learning boom took off in the early 2010s, Nvidia’s stock price has surged as it became obvious that its computer chips were ideally suited for machine learning research and development.
So would an AI revolution in computer graphics be good for the company’s revenue? It certainly wouldn’t hurt, Catanzaro laughs.
“Anything that increases our ability to generate graphics that are more realistic and compelling I think is good for Nvidia’s bottom line.”