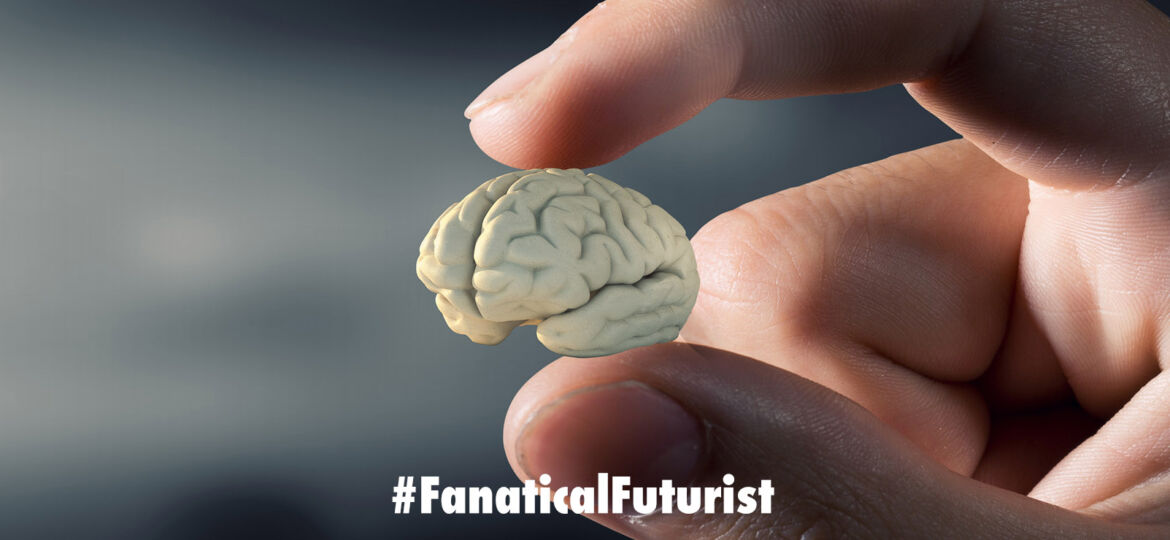
WHY THIS MATTERS IN BRIEF
New computing platforms are getting closer than ever before to being able to pack the power of an entire human brain into a tiny computer chip.
Interested in the Exponential Future? Join our XPotential Community, future proof yourself with courses from our XPotential Academy, connect, watch a keynote, or browse my blog.
The human brain operates on roughly 20 watts of power, or to put it another way, on a third of a 60 watt light bulb, in a space the size of, well, a human head. Meanwhile, the biggest machine learning algorithms use closer to a nuclear power plant’s worth of electricity and racks of chips to learn.
That’s not to slander machine learning, but nature may have a tip or two to improve the situation. Luckily, there’s a branch of computer chip design heeding that call right now and recently researchers in the UK spun up a million core computer that gets us closer to our goal of mimicking the human brain with all its energy efficiencies, in computer form. I am, of course, talking about revolutionary neuromorphic computers. And by mimicking the brain, super-efficient neuromorphic chips aim to take AI off the cloud and put it right in your pocket instead.
MIT’s latest such chip is smaller than a piece of confetti and has tens of thousands of artificial synapses made out of memristors – silicon-based components that mimic the information-transmitting synapses in the human brain.
In a recent paper in Nature Nanotechnology, a team of MIT scientists say their tiny new neuromorphic chip was used to store, retrieve, and manipulate images of Captain America’s Shield and MIT’s Killian Court. Whereas images stored with existing methods tended to lose fidelity over time, the new chip’s images remained crystal clear.
Close-up of the new neuromorphic “Brain-on-a-Chip,” including thousands of memristors, or memory transistors. Credit: Peng Lin
“So far, artificial synapse networks only exist as software. We’re trying to build real neural network hardware for portable artificial intelligence systems,” Jeehwan Kim, associate professor of mechanical engineering at MIT said in a press release. “Imagine connecting a neuromorphic device to a camera on your car, and having it recognize lights and objects and make a decision immediately, without having to connect to the internet. We hope to use energy-efficient memristors to do those tasks on-site, in real-time.”
Whereas the computers in our phones and laptops use separate digital components for processing and memory – and therefore need to shuttle information between the two – the new MIT chip uses analog components called memristors that process and store information in the same place. This is similar to the way the brain works and makes memristors far more efficient. To date, however, they’ve struggled with reliability and scalability.
To overcome these challenges, the MIT team designed a new kind of silicon-based, alloyed memristor. Ions flowing in memristors made from unalloyed materials tend to scatter as the components get smaller, meaning the signal loses fidelity and the resulting computations are less reliable. The team found an alloy of silver and copper helped stabilize the flow of silver ions between electrodes, allowing them to scale the number of memristors on the chip without sacrificing functionality.
While MIT’s new chip is promising, there’s likely a ways to go before memristor-based neuromorphic chips go mainstream. Between now and then, engineers like Kim have their work cut out for them to further scale and demonstrate their designs. But if successful, they could make for smarter smartphones and other even smaller devices.
“We would like to develop this technology further to have larger-scale arrays to do image recognition tasks,” Kim said. “And some day, you might be able to carry around artificial brains to do these kinds of tasks, without connecting to supercomputers, the internet, or the cloud.”
Furthermore, in a recent cost analysis of machine learning, research and investment firm ARK Invest said cost declines in developing and running new AI’s and AI models have far outpaced performance declines in Moore’s Law. In a particular example, they found the cost to train an image recognition algorithm (ResNet-50) went from around $1,000 in 2017 to roughly $10 in 2019. The fall in cost to actually run such an algorithm was even more dramatic – it took $10,000 to classify a billion images in 2017 and just $0.03 in 2019.
While some of these cost declines can be traced to better AI software, according to ARK specialized chips, like the new neuromorphic chips, as well as new and improved GPU’s and specialist AI chips like Google’s TPU processors, have improved in performance by nearly 16 times in the last three years alone, and it’s these developments that have helped improve the cost-performance equation. And if it continues, which it likely will, then soon it will be almost free to develop and run AI’s, and soon we might all be carrying a human brain in our pockets that can do the work of today’s best AI.
Source: MIT