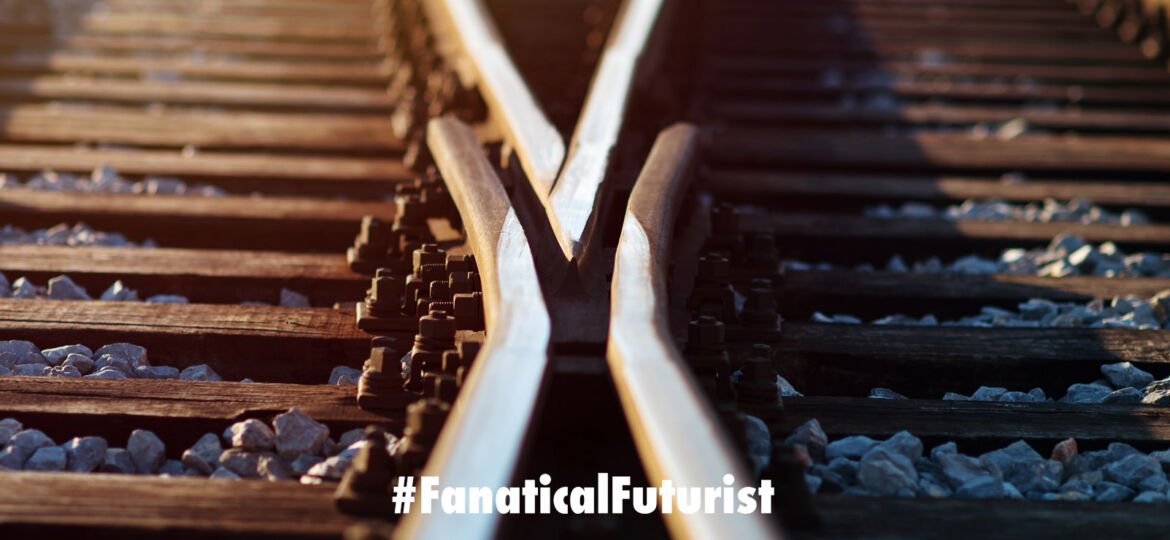
WHY THIS MATTERS IN BRIEF
Humans can’t always predict the outcome of their code and the effect that it will have on decisions, so now AI’s are being given the ability to write their own algorithms.
Love the Exponential Future? Join our free XPotential Community, enjoy premium content, futureproof yourself with XPotential University, connect, watch a keynote, or browse my blog.
When Deep Blue defeated world chess champion Garry Kasparov in 1997, it may have seemed Artificial Intelligence (AI) had finally arrived. After all, a mere computer had just taken down one of the top chess players of all time. But it wasn’t to be.
Though Deep Blue was meticulously programmed top-to-bottom to play chess, the approach was too labour intensive, too dependent on needing to have clearly defined rules and bounded possibilities to succeed at more complex games – let alone in the real world. The next revolution in AI would take a decade and a half, when vastly more computing power and data revived machine learning, an old idea in AI just waiting for the world to catch up.
Today, machine learning dominates, mostly by way of a family of algorithms called deep learning, while symbolic AI, the dominant approach in Deep Blue’s day, has now faded into the background.
Key to deep learning’s success is the fact the algorithms basically write themselves – an increasingly literal statement as we continue to see the rise of so called Machine Programmers – AI’s that design and program themselves, as well as create their own children AI’s, and even dream. Given some high-level programming and a dataset, they learn from experience. No engineer anticipates every possibility in code, so increasingly the algorithms just figure it out by themselves.
Now though, as we see AI’s elsewhere learn to deal with chaos and even evolve themselves, Alphabet’s DeepMind is taking this automation further by developing deep learning algorithms that can handle programming tasks which have been, to date, the sole domain of the world’s top computer scientists and take them years to write.
In a paper recently published on the pre-print server arXiv, a database for research papers that haven’t been peer reviewed yet, the DeepMind team described a new deep reinforcement learning algorithm that was able to discover its own value function, a critical programming rule in deep reinforcement learning, from scratch and without the need for any human intervention which is ground breaking.
Surprisingly, the algorithm was also effective beyond the simple environments it trained in, going on to play Atari games – a different, more complicated task – at a level that was, at times, competitive with human-designed algorithms and achieving superhuman levels of play in 14 games.
DeepMind says the approach could accelerate the development of reinforcement learning algorithms and even lead to a shift in focus, where instead of spending years writing the algorithms themselves, researchers could instead just work to perfect the environments in which they train.