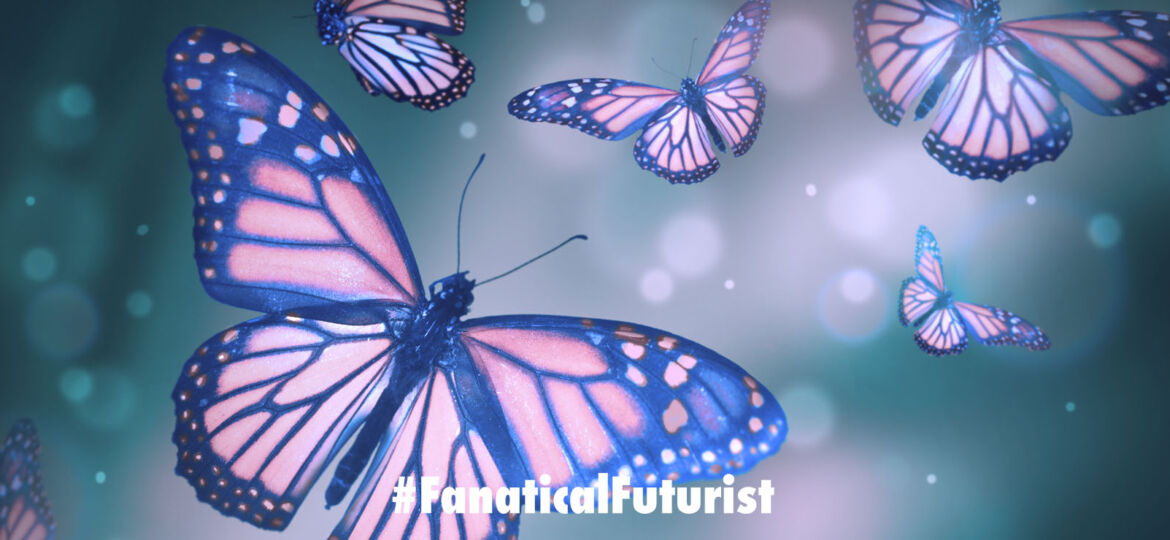
WHY THIS MATTERS IN BRIEF
Life is messy, it’s disordered, it’s chaotic – and so far AI hasn’t been able to get it’s head around it. That’s about to change.
Interested in the Exponential Future? Join our XPotential Community, connect, watch a keynote, or browse my blog.
Artificial Intelligence (AI) is making its way into every corner of our lives, from helping companies determine if you are credit worthy, all the way through to helping discover new COVID-19 vaccines, and help to create better military strategies, but while AI is increasingly adept at multiple things it only really excels at tasks where there’s a rich body of training data that helps it learn what “good” looks like. In short, if it can learn how to perform a task based on historic datasets then it works well. However, if it encounters something it’s never seen before, like “chaos,” then everything quickly goes off the rails which makes building AI models like the US Department of Defense’s KAIROS project which is designed to monitor and predict the effects of chaos around the world, as well as building solid quant investing models that had no idea what investment strategies to suggest when COVID-19 shut the world, so hard.
Neural networks are an advanced type of AI loosely based on the way that our brains work. Our natural neurons exchange electrical impulses according to the strengths of their connections. Artificial neural networks mimic this behaviour by adjusting numerical weights and biases during training sessions to minimize the difference between their actual and desired outputs.
For example, a neural network can be trained to identify photos of dogs by sifting through a large number of photos, making a guess about whether the photo is of a dog, seeing how far off it is, and then adjusting its weights and biases until they are closer to reality. The drawback to this network training is something called “chaos blindness,” an inability to predict or respond to chaos in a system. Conventional AI is chaos blind.
Now, researchers have found that incorporating something called a Hamiltonian function into neural networks better enables them to “see” chaos within a system and adapt their models accordingly.
Simply put, the Hamiltonian embodies the complete information about a dynamic physical system – the total amount of all the energies present, kinetic and potential.
Picture a swinging pendulum, moving back and forth in space over time. Now look at a snapshot of that pendulum. The snapshot cannot tell you where that pendulum is in its arc or where it is going next.
Conventional neural networks operate from a snapshot of the pendulum. Neural networks familiar with the Hamiltonian flow understand the entirety of the pendulum’s movement, where it is, where it will or could be, and the energies involved in its movement.
In a proof-of-concept project, the researchers incorporated Hamiltonian structure into neural networks, then applied them to a known model of stellar and molecular dynamics called the Hénon-Heiles model. The Hamiltonian network accurately predicted the dynamics of the system, even as it moved between order and chaos.
“The Hamiltonian is really the ‘special sauce’ that gives neural networks the ability to learn order and chaos,” says corresponding author John Lindner, a visiting researcher from North Carolina State University and professor of physics at the College of Wooster.
“With the Hamiltonian, the neural network understands underlying dynamics in a way that a conventional network cannot. This is a first step toward physics-savvy neural networks that could help us solve hard problems.”
The work appears in Physical Review E. Partial support for the work came from the Office of Naval Research.
Source: NC State