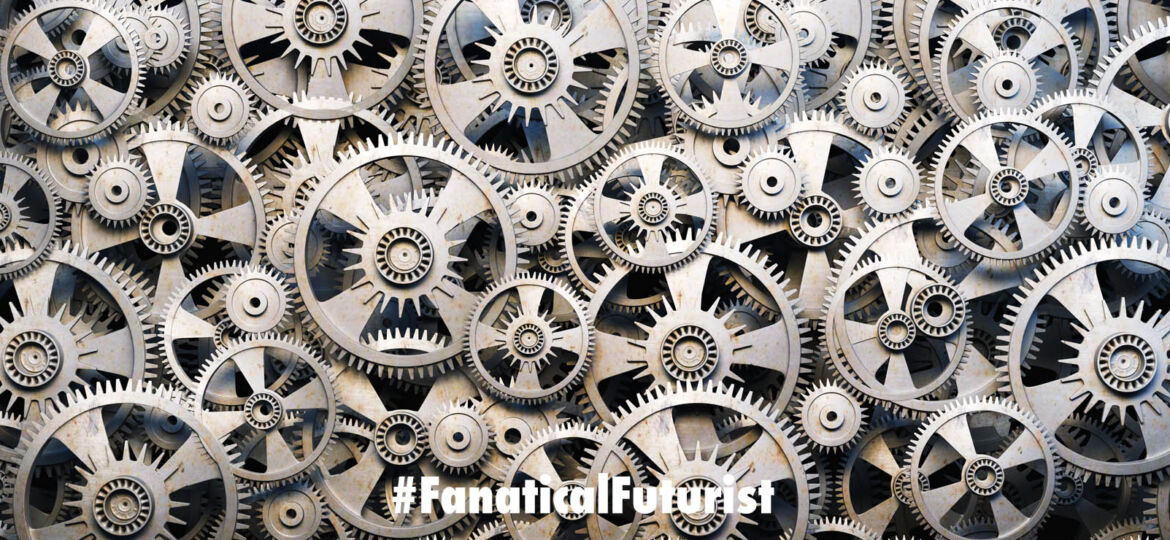
WHY THIS MATTERS IN BRIEF
The ability to use algorithms to create “Architectural Materials” that behave like and mimic artificial intelligence, but in physical form, could revolutionise materials.
Love the Exponential Future? Join our XPotential Community, future proof yourself with courses from XPotential University, read about exponential tech and trends, connect, watch a keynote, or browse my blog.
I’ve seen many kinds of Artificial Intelligences (AI), from ones that are 3D printed to ones that are made of glass and DNA, but now it turns out that a new type of “architected” mechanical material can learn and improve its ability to deal with unexpected forces thanks to a unique lattice structure with connections of variable stiffness, as described in a new paper. The new material gets its properties mainly from the geometry and specific traits of its design rather than what it is made out of.
Take hook-and-loop fabric closures like Velcro, for example. It doesn’t matter whether it is made from cotton, plastic or any other substance. As long as one side is a fabric with stiff hooks and the other side has fluffy loops, the material will have the sticky properties of Velcro.
The Future of Artificial Intelligence, by keynote speaker Matthew Griffin
In this case the team behind the latest innovation based their new material’s architecture on that of an artificial neural network – layers of interconnected nodes that can learn to do tasks by changing how much importance, or weight, they place on each connection.
They then hypothesized that a mechanical lattice with physical connections and “nodes” could be trained to take on certain mechanical properties by adjusting each connection’s rigidity.
To find out if a mechanical lattice would be able to adopt and maintain new properties – such as taking on a new shape or changing directional strength – they started off by building a computer model. They then selected a desired shape for the material as well as input forces and had a computer algorithm tune the tensions of the connections so that the input forces would produce the desired shape. They did this training on 200 different lattice structures and found that a triangular lattice was best at achieving all of the shapes they tested.
Getting up close with the new 2D material
Then, once the many connections are tuned to achieve a set of tasks the material – which is now essentially programmed – reacts in the desired way. The training is, in a sense, remembered in the structure of the material itself like a more traditional memory material.
And now in 3D Form
The team then built a physical prototype lattice with adjustable electromechanical springs arranged in a triangular lattice. The prototype is made of 6 inch connections and is about 2 feet long by 2 feet wide. And it worked. When the lattice and algorithm worked together, the material was able to learn and change shape in particular ways when subjected to different forces. And this is why they decided to call this new material a “Mechanical Neural Network.”
Besides some living materials, very few materials can learn to be better at dealing with unanticipated loads. Imagine a plane wing that suddenly catches a gust of wind and is forced in an unanticipated direction. Most wings, unless they’re made out of metamaterials like this one, can’t change their design to be stronger in that direction.
The prototype lattice material the team designed can adapt to changing or unknown conditions. In a wing, for example, these changes could be the accumulation of internal damage, changes in how the wing is attached to a craft, or fluctuating external loads. Every time a wing made out of a mechanical neural network experienced one of these scenarios, it could strengthen and soften its connections to maintain desired attributes like directional strength. Over time, through successive adjustments made by the algorithm, the wing adopts and maintains new properties, adding each behavior to the rest as a sort of muscle memory.
This type of material could have far reaching applications for the longevity and efficiency of built structures. Not only could a wing made of a mechanical neural network material be stronger, it could also be trained to shape shift into shapes that maximize fuel efficiency in response to changing conditions around it.
So far, they’ve only worked only with 2D lattices. But using computer modelling, they predict that 3D lattices would have a much larger capacity for learning and adaptation. This increase is due to the fact that a 3D structure could have tens of times more connections, or springs, that don’t intersect with one another. However, the mechanisms they used in their first model are far too complex to support in a large 3D structure.
And in terms of what’s next the material they created is a proof of concept and shows the potential of mechanical neural networks. But to bring this idea into the real world will require figuring out how to make the individual pieces smaller and with precise properties of flex and tension.
They also hope new research in the manufacturing of materials at the micron scale, as well as work on new materials with adjustable stiffness, will lead to advances that make powerful smart mechanical neural networks with micron-scale elements and dense 3D connections a ubiquitous reality in the near future.