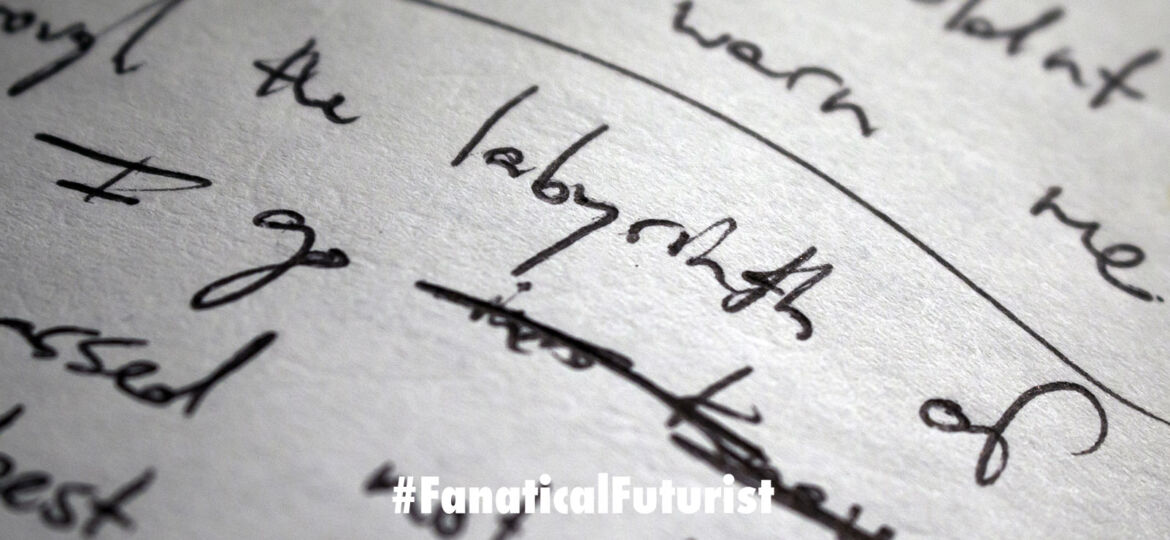
WHY THIS MATTERS IN BRIEF
Most human musicians and composers work by first writing the lyrics, and then the music accompaniment, now machines are copying them.
Interested in the Exponential Future? Connect, download a free E-Book, watch a keynote, or browse my blog.
As the number of creative machines that can generate good synthetic music increases now a new system that uses nothing more than lyrics as the input has appeared on the scene, and thanks to almost daily developments in Artificial Intelligence (AI) the technique might someday become as commonplace as internet radio. In a paper published on the preprint server Arxiv, researchers from the National Institute of Informatics in Japan describe a machine learning system that’s able to generate “lyric-conditioned melodies from learned relationships between syllables and notes.”
“Melody generation from lyrics has been a challenging research issue in the field of AI and music, which enables to learn and discover latent relationship between interesting lyrics and accompanying melody,” wrote the paper’s co-authors. “With the development of available lyrics and melody dataset and [AI], musical knowledge mining between lyrics and melody has gradually become possible.”
As the researchers explain, notes have two musical attributes – pitch and duration. Pitches are perceptual properties of sounds that organise music by highness or lowness on a frequency-related scale, while duration represents the length of time that a pitch or tone is sounded. Syllables align with melodies in the MIDI files of music tracks, the columns within said files represent one syllable with its corresponding note, note duration, and rest.
The researchers’ AI system made use of the alignment data with a Long-Short-Term Memory (LSTM) network, a type of recurrent deep learning network capable of learning long-term dependencies like the human mind, with a Generative Adversarial Network (GAN), a two part neural network consisting of generators that produce samples and discriminators that attempt to distinguish between the generated samples and real-world samples. The LSTM was trained to learn a joint embedding – a mathematical representation – at the syllable and word levels to capture the synaptic structures of lyrics, while the GAN learned over time how to predict melody when it was given the lyrics while accounting for the relationship between lyrics and melody.
To train their new system, the team compiled a data set consisting of 12,197 MIDI files, each paired with lyrics and melody alignment — 7,998 files from the open source LMD-full MIDI Dataset and 4,199 from a Reddit MIDI dataset — which they cut down to 20-note sequences. They took 20,934 unique syllables and 20,268 unique words from the LMD-full MIDI, and extracted the beats-per-minute (BPM) value for each MIDI file, after which they calculated note durations and rest durations.
After splitting the corpus into training, validation, and testing sets and feeding them into the model, the coauthors conducted a series of tests to determine how well it predicted melodies sequentially aligned with the lyrics, MIDI numbers, note duration, and rest duration. They report that their AI system not only outperformed a baseline model “in every respect,” but that it approximated well to the distribution of human-composed music. In a subjective evaluation during which volunteers were asked to rate the quality of 12 twenty second melodies generated using the baseline method, the AI model, and ground truth, scores given to melodies generated by the proposed model were closer to those composed by humans than they were to the baseline.
“Melody generation from lyrics in music and AI is still unexplored well [sic],” wrote the researchers. “Making use of deep learning techniques for melody generation is a very interesting research area, with the aim of understanding music creative activities of human.”
AI might soon become an invaluable tool in musicians’ compositional arsenals, if recent developments are any indication. In July, Montreal based startup Landr raised $26 million for a product that analyzes musical styles to create bespoke sets of audio processors, while OpenAI and Google recently debuted their own online synthetic music systems, with even Sony getting in on the act after developing a machine learning model for conditional kick-drum track generation.