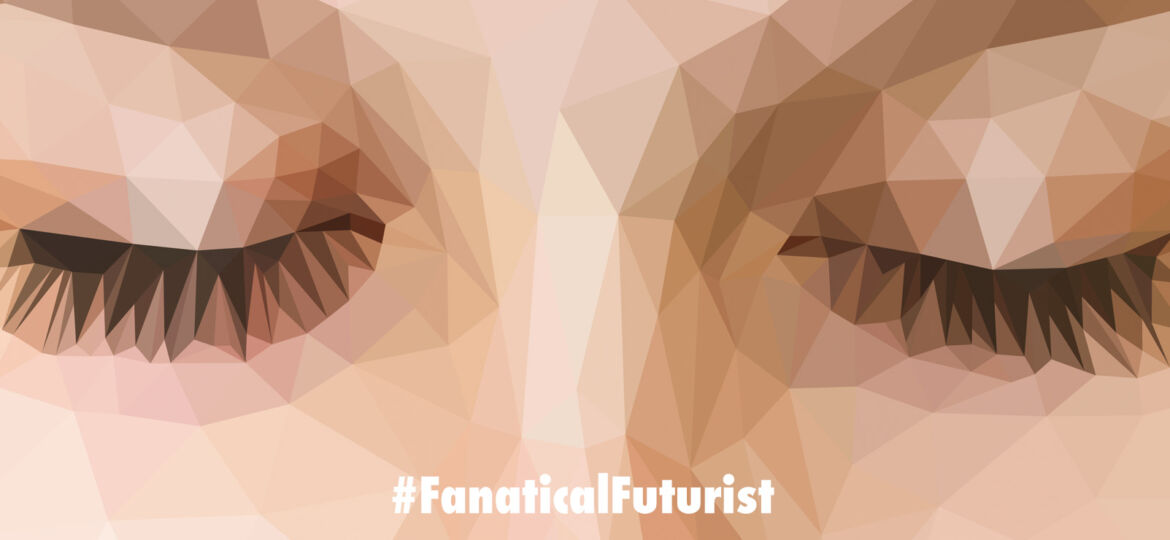
WHY THIS MATTERS IN BRIEF
As AI gets its proverbial head around creating synthetic content the field’s now starting to accelerate, and fast.
Machine Learning is, perhaps, the most common platform for existing Artificial Intelligence (AI) networks. The basic idea is that an AI can be taught to reach its own decisions through exposure to usually huge datasets. It’s similar to how we can learn something by seeing it again and again.
Machine learning algorithms are trained to recognize patterns. For example, a system will be exposed to hundreds, thousands, or even millions of images of cars so it can learn what a car looks like based on characteristics shared by the images. Then, it’ll look for those shared characteristics in a never-before-seen image and determine if it is, in fact, a picture of a car.
While machine learning does an almost perfect job of classifying images, it seems to fumble a bit with generating them. The latest example is an image generator shared as part of the pix2pix project. It’s recently been making the rounds on social media, so I tried it out, and here’s the result…
The end results of the generator are either abstract or hideous, depending on your perspective. But it is undeniably able to turn a simple — and arguably poor — doodle into a far more realistic-looking image.
Like so much of the internet, the pix2pix project started with cats. The same mechanics applied: a user drew an image, and the algorithm transformed it into a (relatively) more realistic-looking cat.
For their generators, the developers used a next-generation machine learning technique called generative adversarial networks (GANs). Essentially, the system determines whether its own generated output (in this case, the “realistic” face) is “real” (looks like one of the images of actual faces from the dataset used to train it) or “fake.” If the answer is “fake,” it then repeats the generation process until an outputted image passes for a “real” one.
The pix2pix project’s image generator is able to take the random doodles and pick out the facial features it recognizes using a machine learning model. Granted, the images the system currently generates aren’t perfect, but a person could look at them and recognize an attempt at a human face.
Obviously, the system will require more training to generate picture perfect images, but the transition from cats to human faces reveals an already considerable improvement. Eventually, generative networks could be used to create realistic-looking images or even videos from crude input. They could pave the way for computers that better understand the real world and how to contribute to it.