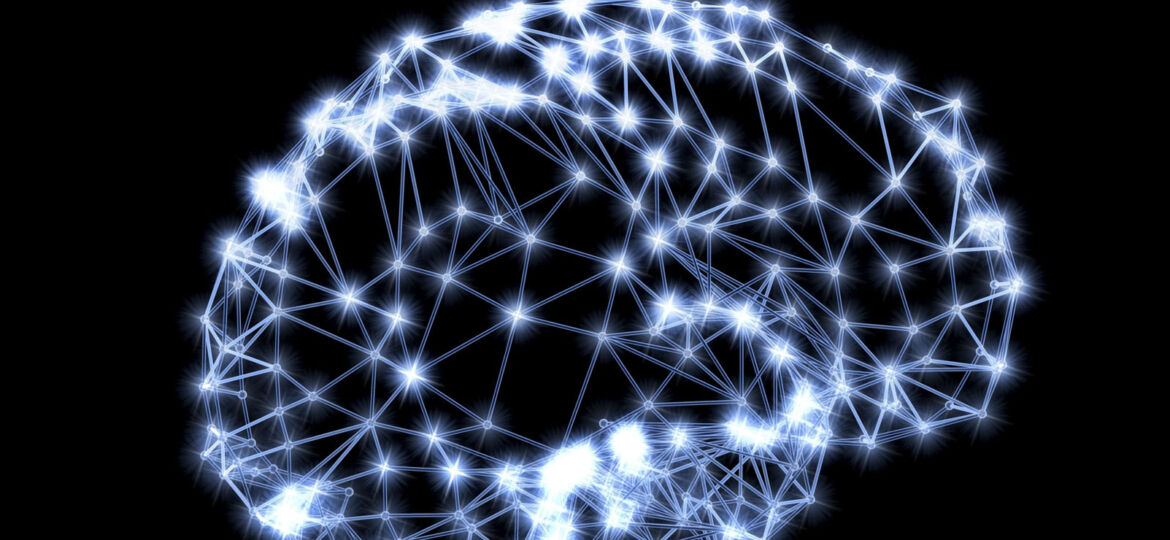
WHY THIS MATTERS IN BRIEF
Memory fills the gaps in information that even todays best AI algorithms can’t.
Google’s DeepMind artificial intelligence lab does more than just develop computer programs capable of beating the world’s best human players in the ancient game of Go. The DeepMind unit has also been working on the next generation of deep learning software that combines the ability to recognize data patterns with the memory required to decipher more complex relationships within the data.
Now, it’s at this point where when we say “memory” you might think that we’re referring to the type of RAM or some other form of hardware computer memory you stick into servers, but no.
We’re talking about mimicking the characteristics of actual human memory. After all, say for example we asked you to predict an outcome then it’s likely that not only will you draw on the information you have on hand at the time but you’ll also – consciously or sub-consciously – draw on past memories that, when accessed and interpreted correctly, will help you create a better, more informed answer – or opinion.
It’s this “human” type memory – this “external” memory as researchers call it – that we’re increasingly seeing being used to augment today’s most advanced deep learning AI’s – such as DeepMind.
Deep learning is the latest buzz word for artificial intelligence algorithms called neural networks that can learn over time by filtering huge amounts of relevant data through many “deep” layers. The brain-inspired neural network layers consist of nodes that architecturally aren’t too dissimilar to our own neurons.
Tech giants such as Google, Facebook, Amazon, and Microsoft have been training neural networks to learn how to better handle tasks such as recognizing images of dogs or making better Chinese to English translations and these AI capabilities have already benefited millions of people using Google Translate and other online services.
But neural networks face huge challenges when they try to rely solely on pattern recognition without having the external memory to store and retrieve information. To improve deep learning’s capabilities, Google DeepMind have created what they call a Differentiable Neural Computer (DNC) that gives neural networks an external memory – that’s not too dissimilar in function to our own – for storing information for later use.
“Neural networks are like the human brain, we humans cannot assimilate massive amounts of data and we must rely on external read-write memory all the time,” says Jay McClelland, director of the Center for Mind, Brain and Computation at Stanford University.
“We once relied on our physical address books and Rolodexes, now of course we rely on the read-write storage capabilities of regular computers.”
McClelland is a cognitive scientist who served as one of several independent peer reviewers for the Google DeepMind paper that describes development of this improved deep learning system. The full paper was presented in the 12 Oct 2016 issue of the journal Nature.
The DeepMind team found that the DNC system’s combination of the neural network and external memory did much better than a neural network alone in tackling the complex relationships between data points in so-called “graph tasks.” For example, they asked their system to either simply take any path between points A and B or to find the shortest travel routes based on a symbolic map of the London Underground subway.
An unaided neural network could not even finish the first level of training, based on traveling between two subway stations without trying to find the shortest route. It achieved an average accuracy of just 37 percent after going through almost two million training examples. By comparison, the neural network with access to external memory in the DNC system successfully completed the entire training curriculum and reached an average of 98.8 percent accuracy on the final lesson. And that is staggering.
The external memory of the DNC system also proved critical to success in performing logical planning tasks such as solving simple block puzzle challenges. Again, a neural network by itself could not even finish the first lesson of the training curriculum for the block puzzle challenge. The DNC system was able to use its memory to store information about the challenge’s goals and to effectively plan ahead by writing its decisions to memory before acting upon them.
In 2014, DeepMind’s researchers developed another system, called the neural Turing machine, that also combined neural networks with external memory. But the neural Turing machine was limited in the way it could access “memories” (information) because such memories were effectively stored and retrieved in fixed blocks or arrays. The latest DNC system can access memories in any arbitrary location, McClelland explains.
The DNC system’s memory architecture even bears a certain resemblance to how the hippocampus region of the brain supports new brain cell growth and new connections in order to store new memories. Just as the DNC system uses the equivalent of time stamps to organize the storage and retrieval of memories, human “free recall” experiments have shown that people are more likely to recall certain items in the same order as first presented.
Despite these similarities, the DNC’s design was driven by computational considerations rather than taking direct inspiration from biological brains, DeepMind’s researchers write in their paper. But McClelland says that he prefers not to think of the similarities as being purely coincidental.
“The design decisions that motivated the architects of the DNC were the same as those that structured the human memory system, although the latter was designed by a gradual evolutionary process, rather than by a group of brilliant AI researchers,” McClelland says.
Human brains still have significant advantages over any brain-inspired deep learning software. For example, human memory seems much better at storing information so that it is accessible by both context or content, McClelland says. He expressed hope that future deep learning and AI research could better capture the memory advantages of biological brains.
DeepMind’s DNC system and similar neural learning systems may represent crucial steps for the ongoing development of AI. But the DNC system still falls well short of what McClelland considers the most important parts of human intelligence.
The DNC is a sophisticated form of external memory, but ultimately it is like the papyrus on which Euclid wrote the elements. The insights of mathematicians that Euclid codified relied on a gradual learning process that structured the neural circuits in their brains so that they came to be able to see relationships that others had not seen, and that structured the neural circuits in Euclid’s brain so that he could formulate what to write. And we have a long way to go before we understand fully the algorithms the human brain uses to support these processes.
It’s unclear when or how Google might take advantage of the capabilities offered by the DNC system to boost its commercial products and services. But Herbert Jaeger, professor for computational science at Jacobs University Bremen in Germany, sees the DeepMind team’s work as a “passing snapshot in a fast evolution sequence of novel neural learning architectures.”
In fact, he’s confident that the DeepMind team already has something better than the DNC system described in the Nature paper – bearing in mind that the paper was submitted back in January 2016 and the field of AI is progressing at an almost furious rate.
DeepMind’s work is also part of a bigger trend in deep learning, Jaeger says. The leading deep learning teams at Google and other companies are racing to build new AI architectures with many different functional modules – among them, attentional control or working memory and they then train the systems through deep learning.
“The DNC is just one among dozens of novel, highly potent, and cleverly-thought-out neural learning systems that are popping up all over the place,” Jaeger says.