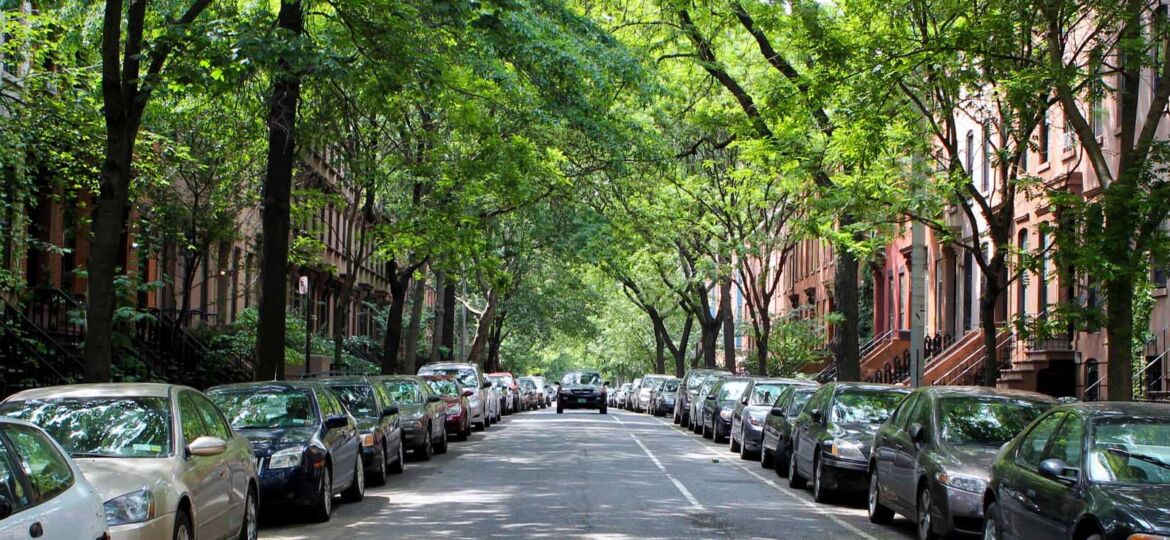
What type of tree is that anyway? Cornell is creating a catalogue for Nature
Engineers from Caltech have developed a method that uses data from satellite and street-level images, such as the ones that you can see in Google Maps, to automatically create an inventory of street trees that cities can use to better manage their urban forests.
“Cities have been surveying their tree populations for decades, but the process is very labor intensive. It usually involves hiring arborists to go out with GPS units to mark the location of each individual tree and identify its species,” says senior author Pietro Perona, at the Allen Puckett Professor of Electrical Engineering in the Division of Engineering and Applied Science.
“For this reason, tree surveys are usually only done every 20 to 30 years, and a lot can change in that time.”
Perona and his team are not expert arborists. Rather, they are leaders in the field of computer vision. They specialise in creating visual recognition algorithms – computer programs capable of “learning” to recognise objects in images – that can see and understand images much like a human would. These algorithms, by replicating the abilities of experts, can sometimes even understand images better than the average person. As part of an ongoing project called “Visipedia,” a collaboration with the Cornell Institute, Cornell University and the Cornell Lab of Ornithology, the engineers have developed algorithms that can recognise the species of a North American bird from a single picture.
The team eventually hopes to develop Visipedia’s capabilities until it can accurately recognise nearly all living things. But they were inspired to turn their attention toward trees when Perona noticed the effects of the years-long California drought on the trees near the Caltech campus in Pasadena.
“I happened to notice that many people in Pasadena were putting drought-resistant plants in their yards to save water, but when they took out the lawns and stopped watering, many trees started dying, and that seemed like a shame,” Perona says.
“I realised that computer vision might be able to help. By automatically analysing satellite and street-level images that are routinely collected, I thought maybe we could carry out an inventory of all the trees and we could see over time how Pasadena is changing, whether the trees that are dying are just a few birch trees, which are not native to California and require frequent watering, or whether it’s truly a massive change.”
To begin their survey of the Pasadena urban tree population, the team developed a method to automatically “look” at any specific location in the city using aerial and street-level images from Google Maps. They then created an algorithm that detects objects within these images and calculates their geographic location. Although a human could easily look at these photographs, spot an object, and ascertain whether or not that object is a tree, the task is not so simple for a computer.
Perona’s research group uses artificial neural networks – algorithms inspired by the brain that allow a computer to “learn” to recognise objects in images but these networks must first receive training from humans.
“We train an algorithm the way you would teach a child – by showing it lots of examples,” Perona says.
“The more examples of trees the algorithm sees, the better it becomes at detecting trees. I must say that a child would learn rather more quickly than our algorithms – right now we need hundreds of examples for each type of tree.”
To provide those examples, the team enlisted some human help via a crowdsourcing service called Amazon Mechanical Turk, in which hundreds of workers worldwide can be quickly recruited to complete simple tasks that require human intelligence. In this case, the so-called “turkers” were asked to look at aerial and street-level images of Pasadena and label the trees in each photo. This information was used to train the algorithm to determine which objects were trees.
The engineers next wanted to train the algorithm to identify the species of each tree in the photos – something that the average person cannot do. Fortuitously, the city of Pasadena had partnered in 2013 with a commercial tree management company called Davey Resource Group (DRG) to complete a tree inventory. The survey included species identification, measurements, and the geographical locations of each of the approximately 80,000 trees in the city. Using this information, the engineers trained the algorithm to identify 18 of the more than 200 species of trees in Pasadena.
From Google Maps aerial and street view images, the engineers obtained four different photographs of each tree in Pasadena, taken from different viewpoints and at different distances from the tree. These photos were then analysed by the algorithm’s “brain” – the artificial neural network. The network then produced a list of a few possible tree species and a score of the certainty of each guess. After comparing the algorithm’s results with those of the 2013 tree survey, the engineers found that their algorithm could detect and identify a tree’s species from Google Maps images with about 80 percent accuracy.
“This was much better than we had expected, and it showed that our method can produce similar results to a tree survey done by humans,” says Steve Branson, a postdoctoral scholar in electrical engineering and coauthor on the paper.
“A human tree expert can identify species at a higher accuracy than our algorithm, but when these large city tree surveys are done they can’t be 100 percent accurate either. You need lots of people to spread out around the city and there will be mistakes.”
Eventually, cities could use Perona’s computer vision software as part of a long-term technological solution for the management of urban forests. The idea is that the software would continuously collect data about urban street trees from satellite and street level images, which are updated every few months, or from other public images. That information then could be incorporated into software that would help the city understand how its urban forests are evolving, and help in the creation of long-term plans for future street-tree investments.
Although perfecting the algorithm is an ongoing process, Perona says the concept could eventually change the way urban forests, as well as one day perhaps, wild forests, are managed, and, frankly after so many companies putting so much effort into intruding on our own privacy it’s about time someone used this technology to help catalogue nature.